The Future of Quantum Machine Learning: Merging Two Frontiers
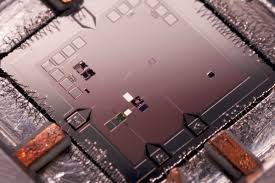
Table of Contents
Quantum machine learning represents a convergence of two groundbreaking fields: quantum computing and machine learning. While both fields have made significant strides independently, their intersection holds immense promise for solving complex computational problems more efficiently than classical approaches. This write-up delves into the potential future of this exciting fusion and how an inclusive Data Analyst Course addresses these two realms of study.
The Future of Quantum Machine Learning
The versatility of combining machine learning and quantum computing are outlines in the following sections.
Quantum Advantage
- Speedup in Computations: Quantum computers have the potential to perform certain calculations exponentially faster than classical computers, particularly for tasks involving large datasets and complex algorithms.
- Enhanced Optimisation: Quantum algorithms could revolutionise optimisation tasks, such as those common in machine learning (for example, parameter optimisation in neural networks), by offering speedups that significantly outpace classical methods. Thus, an inclusive Data Analyst Course curriculum that needs to cover parameter optimisation in neural networks would need to include both machine learning and quantum algorithms.
Algorithm Development
- Quantum Variational Algorithms: These algorithms leverage quantum computing to optimise classical machine learning models, potentially leading to more accurate predictions and faster training times. Up-to-date courses in urban learning centres, such as a Data Analyst Course in Pune or Bangalore often include such emerging topics to address the demand among professionals.
- Quantum Neural Networks: The development of neural network architectures specifically tailored to quantum computing could unlock novel capabilities, such as increased representational power and improved generalisation.
Data Processing and Analysis
- Quantum Data Processing: Quantum computing’s ability to manipulate and analyse large datasets in parallel could enable more efficient data preprocessing and feature extraction. Businesses in commercially active cities, for example, need to handle large volumes of data spread across disparate data sets and would prefer to employ professional who are acquainted with quantum computing, a topic covered in advanced courses such as a Data Analyst Course in Pune or Bangalore tailored for business analysts and strategists.
- Quantum-enhanced Data Analysis: Quantum algorithms may uncover hidden patterns and correlations within data that are difficult for classical methods to discern, leading to more insightful analyses and predictions.
Security and Privacy
- Quantum Cryptography: Quantum computing can bolster security in machine learning applications. Techniques such as the development of quantum-resistant cryptographic protocols that safeguard sensitive data against future threats from quantum adversaries can be learned by attending an advanced Data Analyst Course.
- Privacy-preserving Machine Learning: Quantum techniques might enhance privacy-preserving machine learning methods by providing secure multiparty computation and homomorphic encryption protocols that are resistant to quantum attacks.
Experimental Progress
- Real-world Applications: Continued experimental progress in quantum computing hardware and software will pave the way for practical applications of quantum machine learning in various domains, including drug discovery, finance, and materials science.
- Hybrid Approaches: Hybrid quantum-classical algorithms, combining the strengths of both paradigms, are likely to emerge as a pragmatic approach for leveraging near-term quantum devices to solve real-world machine learning problems.
Challenges and Limitations (Quantum Machine)
- Hardware Constraints: Overcoming noise, errors, and scalability limitations in quantum hardware remains a significant challenge for realising the full potential of quantum machine learning algorithms.
- Algorithmic Development: Developing quantum machine learning algorithms that outperform classical methods across a broad range of tasks requires substantial theoretical and empirical research. This is a core technology that draws from both machine learning and quantum computing and a highly preferred topic in a professional Data Analyst Course.
- Integration with Classical Systems: Bridging the gap between quantum and classical computing architectures is essential for seamless integration of quantum machine learning techniques into existing computational workflows.
Summary (Quantum Machine)
In summary, the future of quantum machine learning holds great promise for transforming the landscape of artificial intelligence and computational science. By harnessing the unique properties of quantum systems, researchers aim to unlock unprecedented capabilities for solving complex problems and driving innovation across diverse fields.
Contact Us:
Name: ExcelR – Data Science, Data Analytics Course Training in Pune
Address: 101 A ,1st Floor, Siddh Icon, Baner Rd, opposite Lane To Royal Enfield Showroom, beside Asian Box Restaurant, Baner, Pune, Maharashtra 411045
Phone Number: 098809 13504
Email ID:shyam@excelr.com